CASE STUDY
Mining of social health networks reveals early and undetected chemotherapeutic adverse drug reactions
What can online social health networks reveal about chemotherapy adverse drug reactions?
Cutaneous adverse drug reactions (CADRs) occur in many patients who receive chemotherapy. Also known as toxidermia, CADRs range from mild skin lesions to potentially life-threatening toxic epidermal necrolysis (TEN) or Lyell’s syndrome.
Clinical trials don’t always detect CADRs, and the literature is often late in reporting these adverse events. Millions of patients often turn to online social health networks to seek advice on health concerns like CADRs. These real-time, online health conversations provide an opportunity to detect CADRs early. Early detection may significantly reduce morbidity and healthcare costs. Unfortunately, pharmaceutical companies tend to underutilize information shared on social health networks. As a result, they miss an opportunity to strengthen post-marketing surveillance.
To accelerate the earlier detection of chemotherapeutic CADRs, Inspire researchers partnered with physicians and researchers to conduct a study using a natural language processing-based signal-generation pipeline. This methodology enabled the research team to accurately detect patient reports of CADRs more than seven months in advance of literature reporting.
Research findings, published in the April to June 2019 issue of the Journal of Medical Internet Research (JMIR), reflect the important role that social health network data can play in the early detection of unreported and underreported aftermarket adverse drug events (pharmacovigilance).
Project focus: Evaluate the effectiveness of a natural language processing-based signal-generation pipeline for the early detection of CADRs
The research team created a natural language processing-based signal-generation pipeline to analyze more than 7 million Inspire member posts shared across numerous online health communities. The goal was to see if there was an association between specific targeted cancer therapy drugs and CADRs, as reported by Inspire community members.
Accessing this type of real-time conversational analysis can help pharmaceutical and healthcare clients identify drug side effects that traditional clinical trials might not detect. This information offers drug companies an opportunity to educate healthcare providers and their patients about potential medication side effects. Physicians can use this information to help their patients understand and manage drug adverse reactions.
Inspire solution: Use deep health mining of online community posts to identify chemotherapeutic adverse drug reactions
Researchers focused on two classes of chemotherapies: epidermal growth factor receptor (EGFR) inhibitors and immune checkpoint inhibitors. In use for more than 15 years, EGFR inhibitors have well-established, known side effects. This study focused on a particular EGFR called erlotinib. Researchers identified 55,778 Inspire community posts that mentioned erlotinib.
The study focused on two immune checkpoint programmed cell death 1 (PD-1) inhibitors: nivolumab and pembrolizumab. The Food and Drug Administration (FDA) approved these cancer treatments in 2014, so data about their side effects is less robust. Researchers identified 15,738 online posts mentioning nivolumab and pembrolizumab.
The research team employed user-generated content to identify more than 7 million discussion posts about these three drugs shared by Inspire community members from 2005 to 2016. To retrieve relevant drug-related posts, the team employed regular expressions, a simple text-processing method that implemented the exact string match. They also defined a set of eight common and rare chemotherapeutic CADRs to study.
Steps for mining health networks for key data
Other research steps included:
- Using DeepHealthMiner (DHM), a neural network-based named entity recognition system trained to extract drug safety-related data from user-generated social media content.
- Retraining DHM to use labeled and unlabeled posts from Inspire by adding the more than 7 million Inspire community member posts.
- Creating a subset of 200 Inspire posts that contained keywords from rare CADRs.
- Evaluating the performance of the retrained DHM by creating an Inspire test set of 50 randomly selected posts.
- Storing extracted CADR mentions and drug-related information in a database for further analysis.
- Defining a lexicon of eight common and rare chemotherapeutic CADRs to study.
- Building an Apache Lucene™ repository by creating one Lucene document for each lexicon entry.
- Indexing for Unified Medical Language System (UMLS) Concept Unique Identifiers (CUIs).
- Retrieving a ranked list of the matched, relevant CADR concepts and selecting the top-ranked concept from the list.
- Calculating and calibrating the proportional reporting ratio (PRR) to quantify the associative strength between a CADR and drug.
- Validating drug-CADR pairs with known and common associations having PRR values greater than 1 (user posts having at least one drug mention).
Identifying 13,600 EGFR concepts and 812 immune checkpoint inhibitor concepts mapped to the eight CADRs.
Inspire findings: Online forums can reveal underreported and novel adverse drug reactions
There were two key takeaways from this study:
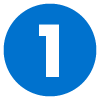
Earlier detection of CADRs
This study demonstrated the potential of extracting information from online social media forums to detect adverse drug reactions earlier than the literature. The pipeline system was able to detect patient-reported CADRs an average of seven months earlier than literature reporting. The analysis reflected an equally high precision (0.90) of CADR reports and at similar frequencies as those found in the literature.
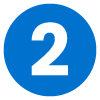
Detection of novel CADRs
The system also identified a never-before-reported CADR (hypohidrosis or inadequate sweating) associated with erlotinib. There were 23 unique Inspire community members reporting hypohidrosis in association with erlotinib as early as 2006. This adverse drug reaction has been absent from the literature since the drug’s development. Researchers shared this novel finding in an April 2018 JAMA Oncology research letter.
This study further validates the use and importance of natural language processing-based signal-generation pipelines in pharmacosurveillance. Researchers can adapt the research methods employed in this single-generation pipeline to other drugs and side effects with high precision. Pharmaceutical and healthcare clients can benefit from mining online patient forums to help identify post-market adverse drug reactions.
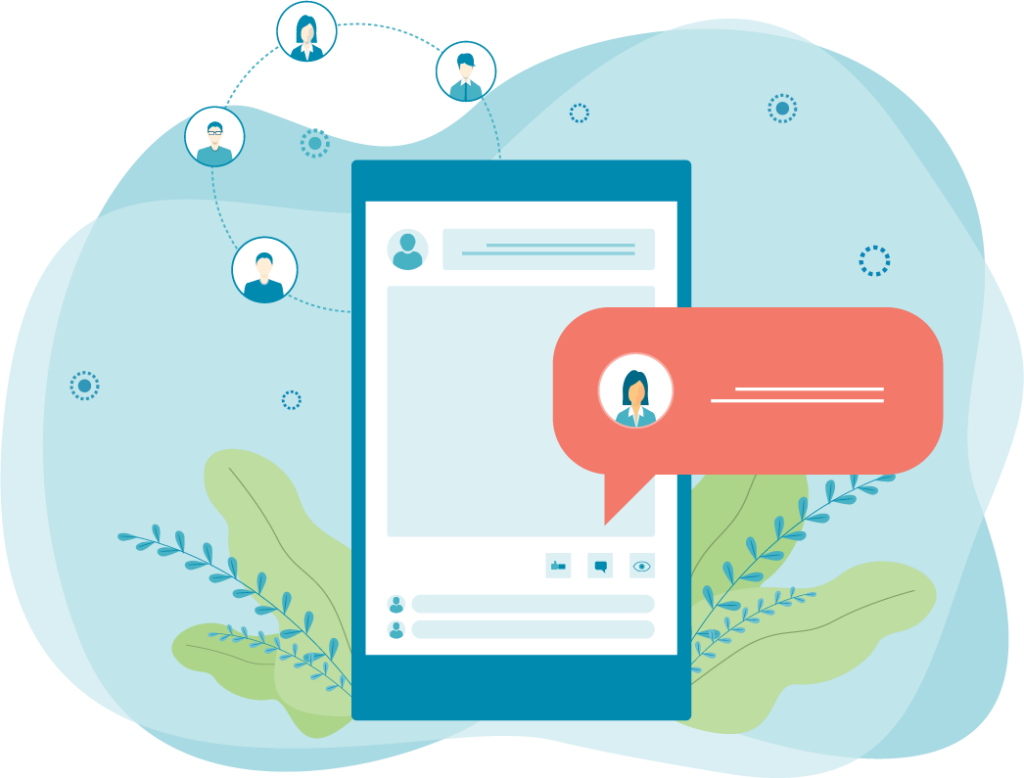
Contact Inspire
"*" indicates required fields